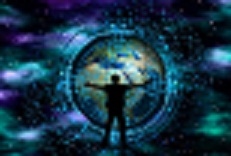
Chain of Thought Prompting: The Future of AI Reasoning
The world of AI is now running at speeds unprecedented. Of the various key areas in the realm of AI breakthroughs, NLP happens to be among the most vital areas where machines can be empowered to better comprehend and converse like humans. Among one of the highly influential techniques developed over the past few years has been chain of thought prompting. Chain of thought prompting has been recognized as the future big leap for enabling machines to solve complex problems. After a technique that improves the capabilities of LLMs in reasoning like GPT, this paper below describes what chain of thought prompting is and how it works and what this might mean for the future of AI.
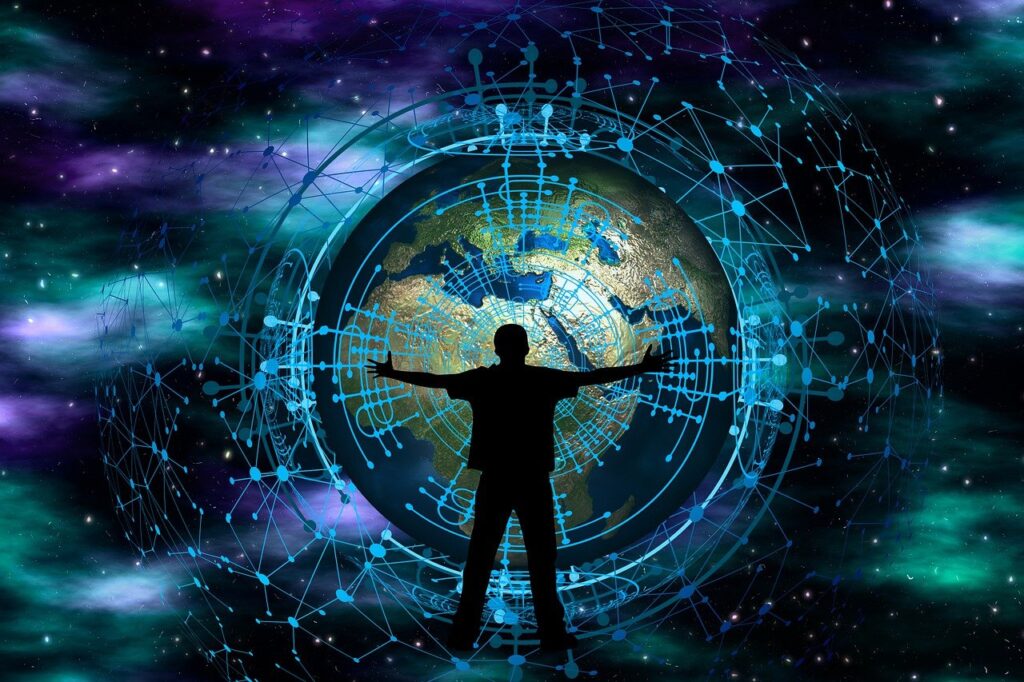
Image of Chain of Thought Prompting, Image designed by Pixabay
What is Chain of Thought Prompting?
Chain of thought prompting encourages the reasoning abilities of LLMs by nudging the model to think stepwise, as we all do when solving a complex problem. The model will produce the working steps toward the final answer, which sounds similar to what we have in the way we structure our problem-solving when we have to answer a complex math problem, plan a project, or make a decision.
For example, for this mathematical problem: “A store sells apples at $2 each and bananas at $3. How much will it cost to buy 3 apples and 2 bananas?” Chain of thought prompting from the AI would break this down this way:
1. The 3 apples are going to be 3 $2= $6
2. Two bananas add up to $6.
3. Cost total: $6 + $6 = $12
This step-by-step reasoning works out to a much more accurate and interpretable answer.
Why Chain of Thought Prompting Matters
LLMs are extremely powerful but weak at complex reasoning tasks. The traditional model excels at tasks that demand short, pointed answers but breaks down when faced with multi-step problems or questions requiring logical deductions. Chain of thought prompting bridges this deficiency by improving the model in solving various areas:
– Math word problems
– Questions which require multi-step reasoning
– Logical deductions.
– Contextualization to retain information during several steps.
This technique not only enhances the model to be more correct but also makes the reason-giving mechanism evident. It’s a technique through which users will know how the AI derived the result as it’s going to be an explainable, step-by-step mechanism so that there would be trust and accountability.
Chain of thought prompting relies on two primary factors: the design of the prompt and the internal properties of the language model.
1. The Design of the Prompt.
This model’s user or developer must feed it some examples illustrating how it applies step-by-step reasoning. Those examples are engineered in such a way that the model is pointed to output relevant answers for new, unseen questions. For example:
– Q: There are 3 red balls and 5 blue balls in a box. If 2 red balls and 1 blue ball are taken out, then how many remain?
A:
– Step 1: Total balls = 3 (red) + 5 (blue) = 8.
– Step 2: Balls taken out = 2 (red) + 1 (blue) = 3.
– Ball remaining = 8 − 3 = 5.
That is, the model learns how to generate such a process of structured reasoning while giving it that kind of example.
2. Model Capabilities
Chain of thought prompting is the most powerful with fine-tuned LLMs over large logical and mathematical content rich datasets. Models such as GPT-4, PaLM (Pathways Language Model) and others can process these prompts more effectively due to the understanding of patterns, logic, and structure.
Applications of Chain of Thought Prompting
It will revolutionize how AI systems interact with data, solve problems, and assist users across many domains. The applications of this type of chain of thought prompting include the following:
1. Education and Tutoring
Chain of thought prompting can be implemented in AI-tutoring for teaching purposes to students. Using AI tutors breaks down the math problems or concepts in science that are otherwise highly complex into relatively easier steps so that learning occurs more easily and effectively.
2. Medical Diagnosis
In healthcare, an AI system would employ chain of thought reasoning for use by physicians to diagnose illnesses. The AI system can present symptomatically followed test results, a patient history that leads in a logical succession to possible diagnoses and potential treatments.
3. Legal Analysis
Chain of thought prompting can break a complex legal issue into steps that are easy to handle. For instance, with AI, one can smoothen the analysis of case laws, statutes, and precedents into forming legal arguments.
4. Finance and Business Analytics
Chain of thought prompting will help businesses in making sense out of their financial data, risks, and even generating insights. For instance, an AI could break down, step by step, revenue trends, cost structures, and market conditions as guides in decision-making.
5. Scientific Research
Researchers often come across problems that are complex and demanding logical reasoning or hypothesis testing. Chain of thought prompting can analyze experimental data to draw conclusions, even suggest further experiments.
Chain of thought prompting is a revolutionary technique, but it is not without challenges:
1. Model Dependency
The success of chain of thought prompting will depend on the capabilities of the LLM. Less advanced models have a harder time actually producing accurate step-by-step reasoning.
2. Prompt Engineering Complexity
Effectively designing chain of thought prompts is very resource intensive. Badly designed prompts often lead to erroneous or incomplete answers.
3. Computation Overhead
More computation than is required for a direct answer is needed to provide step-by-step explanations. Such may be too expensive for a real-time system or other computational devices with processing power constraints.
Future of Chain of Thought Prompting
Such chains of thought inducing signalling marks a new milestone in the attempts of AI systems to reason within the system, and with advancing AI, further improvement will see innovative techniques combined with potential new avenues such as:
Chain of Thought Generation Dynamic: Dynamics allowing a change in the current process of reasoning to adapt in real-time cycle and feed back the user with real-time changes to the situation.
Multimodal Reasoning: Coupling chain of thought prompting to include visual, auditory, or other forms of data in the problem-solving procedure, cutting across several domains.
Improved Interpretability: Tools to make the model’s reasoning process easier to visualize and analyze, which can help users better understand and trust AI systems.
Chain of thought prompting is the new revolution in the capabilities of AI reasoning. AI systems are allowed to solve intricate problems, make more accurate determinations, and gain the confidence of the users through transparency through mimicking human-like logical processes. Challenges persist; however, chain of thought prompting promises to bring unprecedented applications across areas such as education, healthcare, finance, and many others.
With improvements to this method and its implementation into the rapidly emerging AI technology, chain of thought prompting is sure to become the basis of future AI systems. Not only will it improve the ability of machines but bring us one step closer to that dream of thinking, reasoning, and problem-solving AI.